ProxylessNAS: Direct Neural Architecture Search On Target Task And Hardware
Problems To Solve
- 기존 cotroller를 사용하는 방식의 NAS는 많은 GPU Time이 걸렸다.
- 이를 해결하기 위해 NAS의 objective function을 differentiable하게 만들어 archtecture search를 한 시도는 GPU Time은 많이 줄일 수 있었으나 GPU Memory 사용이 많았다.
- 더불어 ImageNet과 같은 큰 데이터셋에 대해서는 시간이 오래 걸려 proxy를 사용하여, network를 evaluation을 하려는 시도가 있었으나 proxy task에 evaluation된 아키텍쳐가 target task에서 성능도 좋을 것이란 걸 보장하지 못했다.
*proxy tasks: training for fewer epochs, starting with a small dataset, or learning with fewer blocks
Method to Solve Problem
1. Construction of Over-Parametrized Network
$$ \mathcal{N}(e_{1},\cdots,e_{n}) \; where \; e_{i}: edge \; in \; DAG $$
$$\mathcal{O} = {o_{i} }:set \; of \; N \; candidate \; primitive \; operations \$$
$$\mathcal{N}(e=m_{\mathcal{O}}^{1},\cdots,e_{n}=m_{\mathcal{O}}^{n}):over-parametrized \; network$$
-
위와 같이 Over-parametrized network를 구성했다. 여기서 mOmO는 N개의 parallel paths를 갖는 mixed operation을 나타낸다.
-
mixed operation의 출력은 One-Shot과 DARTS에서 달리 정의되었는데 이는 아래와 같다.
-
식에서 볼 수 있듯이 N개의 paths에 대해 output feature maps을 모두 계산하고 메모리에 저장해야되기 때문에, 하나의 path만 사용하는 compact model 대비 학습시 GPU memory/hours가 N배 차이난다. ImageNet과 같은 large-scale dataset에 대해서는 memory limit을 쉽게 초과할 수 있어 memory consumptuion을 줄이기 위한 path binarization이라는 아이디어를 제시했다.
*식에서 나오는 αiαi는 N real-valued architecture parameter를 나타낸다.
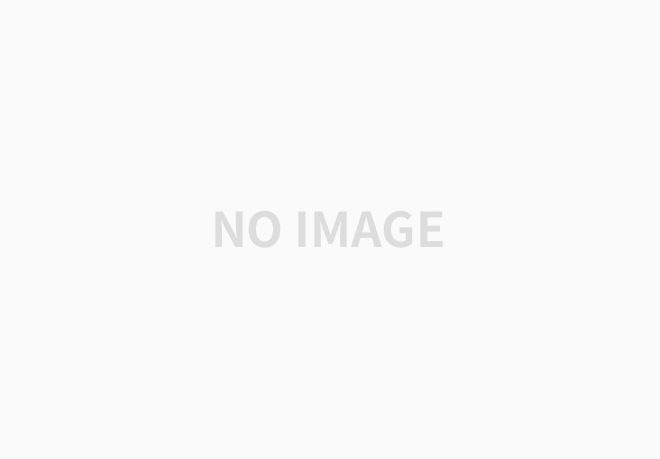
2. Learning Binarized Path
-
Memory footprint를 줄이기 위해 over-parametrized network 학습 시에 하나의 path만 두었다고 한다.
-
이때 N real-valued architecture parameters $ {\alpha_{i} } 를 두고, real-valued path weights을 binary gates로 변환시켰다.
-
binary gate g를 사용하였을 때 output of mixed operation은 다음과 같다.
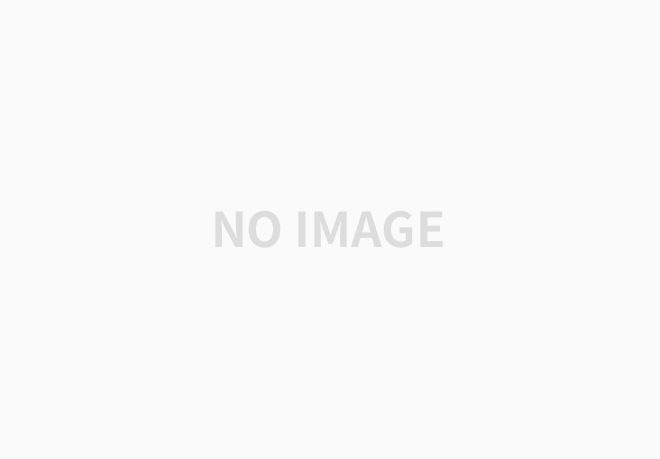
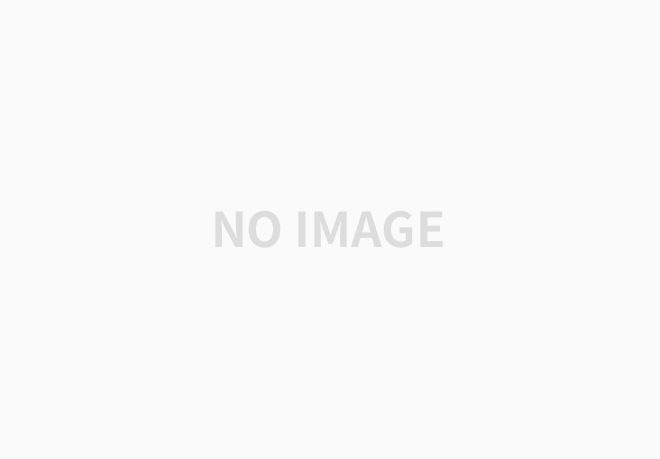
2.1 Training Binarized Architecture Parameter
Training procedure
- weight parameter를 학습시킬 때는 architecture parameter를 freeze 시키고 binary gates를 샘플링한다.
- weight parameter를 training set에 gradient descent를 사용하여 업데이트 했다.
- architecture parameter를 학습시킬 때는 weight parameter를 freeze시키고 binary gate를 reset한다.
- architecture parameter를 validation set에 gradient descent를 사용하여 업데이트했다.
- architecture parameter 학습이 끝난 후, redundant paths를 pruning했다.
- 논문에서는 가장 높은 path weight을 택했다고 한다.
- architecture parameter가 computation graph에 포함되어 있지 않기 때문에 gradient descent로 업데이트가 불가능하기 때문에 이를 학습하기 위한 gradient-based method를 소개했다.
3. Handling Non-Differentiable Hardware Metrics
- 정확도 뿐만 아니라 latency는 하드웨어를 고려하여 효율적인 뉴럴넷을 설계할 때 중요한 목표가 된다.
- 하지만, loss function의 gradient를 사용하여 정확도를 최적화할 수 있는 것과 달리 latency는 미분 불가능하기 때문에 최적화하기 힘들다. 논문에서는 non-differentiable objective를 다룰 수 있는 두 가지 접근법을 제안했다.
3.1 Making Latency Differentiable
-
latency를 미분가능하게 만들기 위해 network의 latency를 뉴럴넷 차원의 연속함수로 모델링했다.
-
path weight: pjpj, candidate set: ojoj라고 했을 때 mixed operation(learnable block)의 expected latency를 다음과 같이 정의했다.
E[latencyi]=∑jpij×F(oij) -
좌항은 i번째 learnable block의 expected latency를 나타내고, F()는 latency prediction model을 나타낸다.
-
이를 architecture parameter에 대해 gradient를 계산하면 다음과 같다.
∂E[latencyi]∂pij=F(oij) -
이를 모든 learnable block에 대해 계산하여 합친 것은 network의 inference latency로 보았고, 이를 loss function에 정규화항으로 넣었다.
3.2 Reinforcement-based Approach
- 논문 참고
'연구 > 논문 리뷰' 카테고리의 다른 글
[NIPS 2017] Attention Is All You Need (0) | 2020.10.24 |
---|---|
[NIPS 2020] Pruning Filter In Filter (0) | 2020.10.07 |
[Arxiv] Single Shot Structured Pruning Before Training (0) | 2020.10.03 |
[ICLR 2020] Once for All: Train One Network and Specialize it for Efficient Deployment (0) | 2020.08.05 |
[ECCV 2018] ShuffleNet V2: Practical Guidelines for Efficient CNN Architecture Design (0) | 2020.06.15 |